Semantic search is a search behavior that relies on the meanings of concepts, words, and sentences. Semantic search is a mutual feature between semantic search engines and semantic searches. Semantic search sessions involve understanding the meaning of every word, and sentence in web documents and queries based on human language and neural nets. Semantic web and Semantic SEO derive from semantic search.
Semantic search behaviors are different from lexical search behaviors. Lexical search behaviors try to use more robotic queries, and explicit contextual-related terms, while semantic search uses more patterned behaviors based on natural language. Google stated that every year, queries have more and more natural language queries. Natural language queries are queries that use daily-life normal, and routine language. Tim-Barnes Lee stated that the semantic web is the web that uses semantic behavior patterns. And, these semantic behavior patterns come from human nature. Semantic search uses natural language queries with these behavior patterns. The Association of things in knowledge representation comes in different methods like “frames”, “semantic networks”, “production rules”, “E-A-V”, or “knowledge bases”. These association networks in knowledge representation help search engine optimization. And, most of the association networks are derived from the “predicates”, or the “things that we do in daily life” as stated in Semantic Search and semantic behavior patterns. “To behave”, you need a “verb from human perception”.
The semantic Search for Semantic SEO guide involves an SEO Case Study from a Semantic Content Networks article. This article involves two different websites. Since the topic is heavily advanced and exhausting, I decided to create two more helpful articles to expand these topics. In this context, the second website sample from the “Semantic Content Networks” SEO Case Study which is “Istanbulbogazicienstitu.com” will be explained from a different perspective, but more practically.
To understand the Semantic Content Networks further, read the previous, “Semantic Content Networks” article that explains the first website sample.
The specific case study is written on 26 May 2022. But, it is updated with the new projects and examples at the bottom.
Why do the Semantic Search Algorithms of Google require Military Discipline? Military discipline doesn’t lose its routines and reflexes after achieving success. Some of the SEO Projects that I published with a high level of success by acquiring topical authority lose their discipline, and start to distort the topical map that I created. This happens because usually, for monetization, they open new pages for small queries, or they break the website information tree, by replacing the specific entities, and by deforming the distances and associations between entities, and contexts. They decrease the publication quality by removing the search engine trust that comes from the previously published content. To prevent these types of problems, I would suggest you learn from the SEO Case Studies in this specific map.
The Initial Results and the Updated Version of the Sample Case Study Website
The Semantic Content Networks SEO Case Study has been deepened by examining the ranking algorithms of the search engine. “How Google Ranks” research helps to understand how Google reacts to the changes on its websites. Below, you will find the initial results of the SEO Case Study.
This first jump happened during a “seasonal SEO event” which was measured and used for increasing a specific website’s relevance and authority for certain topics.
The first and second screenshots have only 15–20 days of a break between them. The increase has continued with new queries, rankings, and organic search performance.
The SEMrush showed the specific website’s organic ranking as above. Below, you can see the updated version after 2 months.
The query gains changed as below.
Below, you can see the updated version of the current situation from Ahrefs.
I have said that the only things I have put on this website are “two different semantic content networks.” And, it was highly painful with the team of authors of the client. Thus, I didn’t publish the third one, but in May, probably, I will start publishing the third one.
This article focuses on finding the “predicate-connected” associations between different patterns and semantic behaviors.
Semantically Connected Predicates from the Life of a Human, or Collection of Humans
Semantic SEO requires two main features for a consultant or expert.
- Understanding search engines.
- Understanding the philosophy of knowledge
This article focuses more on the second section to explain how to shape semantic content networks.
Let’s write a few verbs below to understand the different types of connections between them.
- Work
- Study
- Master
- Investigate
- Learn
- Improve
- Increase
The verbs above are connected to each other, in fact, you can even use certain types of “nouns” mutually for nearly all of them.
Such as “working on X”, “studying X”, “examining X”, “investigating X reasons” “learning X types, “Improving X efficiency”, and “Increasing X effects”. X can exist for all these verbs. Furthermore, all the outcomes of the X and X-related verbs can be linked to some other related concepts and verbs. Some of these connected verbs with different nouns and their phrasification versions are below.
- Earn
- Deserve
- Make
- Have
- Collect
- Gain
- Acquire
- Secure
For all of these verbs, we can write some different “nouns”, or nouns that are nearly the same. For example, “earn money”, “deserve raise”, “make money”, “have more money”, “collect money”, “gain money”, “acquire profit”, “secure profit”, “deserve financial freedom”, “deserve raise” and more.
These two different verb groups have many common things between them. And, if you think that the language algorithm BERT has been trained over 3.5 billion words, and word connections, it is more and more possible. The BERT Language Algorithm works based on word sequence. For example, if you change the previous predicate to “function”, the object will be “with word sequences”. These verbs and their mutual contexts, or central words with centralized connections, help in shaping the semantic content networks based on the semantic search.
Certain predicates have certain types of semantic context signifiers such as “examine” signals the “reason”, and “increase” signals the amount, or intensity. Thus, the “objects” and their “attributes” are signaled by the predicates, as in triples and Entity-Attribute-Value Models. The E-A-V Models and SEO connection have been explained further in the “Choosing the Right Attributes with Accurate Values from Text” SEO Case Study. Especially, for this specific project, the “Creating Semantic Content Networks SEO Case Study”, it is stated that due to the author’s issues, the third semantic content network is not published, and it can affect the website negatively in the future. Since the website couldn’t complete the Frames of Predicates with possible attribute and object connections by processing every contextual domain, it can be replaced by being labeled as a half-authority source. Thus, it shows the importance of discipline and consistency for the Holistic SEO approach. The possible problems for semantic content network creation are explained in the Expanding Topical Maps SEO Case Study.
The related sections and warnings for the third semantic content network are below.
Verbs, and Connected Semantic Behaviors
Even if I had written all these verbs and their possible nouns without stopping, and randomly, the connection between them would have naturally emerged. Because I am a semantic creature/biological existence. In other words, another chance wouldn’t be possible. If you do not let your brain forget the previous word, it will find a relevant word for you. That’s why Neural Networks and Deep Learning are related to your brain. The human brain works with connections, association, and denotation. Your words follow each other based on your latest memory sample for that word. If you focus on meaning, it changes. For example, the word “horse” might make you remember a specific thing, but if you use the same concept in Spanish, the word might make you remember the Spanish teacher from your university. In this context, when 999 million people have searched for “X” with 999 million different backgrounds, a semantic search engine needs to understand it. Do they mean “horse in Spanish”, “horse speed”, “horse anatomy”, or something else… These semantic content networks cover every possibility of word connections based on the search engine’s understanding, and all these words’ contexts come from semantic search behaviors and their patterns.
The verb and noun connections like “improve a skill”, “increase the profit”, or “earn money” and “learn a skill” are connected to each other through the same semantic search patterns. You can generate, 99,999,999 different combinations of verbs and nouns that have the same meaning.
Sculpting the Context for Deepening and Sharpening it
Context deepening and sharpening are stated and processed before in the Contextual Search Engine article that I have written. When you have multiple different verb and noun connections, and combinations of connections, you need to refine them further only for a certain area.
Earning money, or improving the efficiency of a process, are too vast contexts. There might be millions of queries, or possible search intents and behaviors semantically, for all these word sequences. Thus, for a semantic content network, a Source Context is a must. What is the purpose of the brand? Whom should the brand reach out to with its own content network? From these answers, the context might be sharpened and deepened.
If the context is for restaurants, “make money from the best sauces,” “increase profit with the best customer relationships”, “learn new food trends in the US”, “deserve more positive food service reviews from clients”, or other types of verbs and noun chains can be used.
If the context is for students, “make money online”, “make money while studying”, “learn new skills”, “improve your reading speed to study faster,” or any other connected things can be found. But, always “learn” and “earn” will be the main central predicates for creating all these semantically relevant content networks.
Another important step here is “canonicalization”. Even if the context is deepened or shaped, still the specific examples are still too vast, and not “query-focused”. Search engines always compare a query to a document, thus, vocabulary coverage between the document and the query is a must.
Query-focused Semantic Vocabulary Configuration
The vocabulary of the query and the web documents should align with each other. Query-focused semantic vocabulary configuration involves including the query terms while canonicalizing the verbs and nouns based on the specific query characteristics. If the query contains a certain pattern, the verbs that are included in the queries can be replaced by each other, because the search engine will index all these related web documents based on the triples, of object, predicate, and subject. In the Cost of Retrieval SEO Case Study, all these word connections, and the entity-indexation process are explained further. A verb, a noun, and a query pattern can create a document template. Thus, the semantic content networks SEO case study focuses on “query templates”. A semantic search engine can focus on the query templates rather than the individual query perception, it helps a search engine to connect the search behaviors better. And, every “behavior” comes from a verb. Thus, understanding the verb forms of life is a must to create probable probabilities and their connections.
The query-focused semantic vocabulary configuration is a must to create a proper query-focused context consolidation. The verbs can be synonyms based on their subjects. “Running a business”, and “functioning in a workplace”, the verbs “run” and “function” are not synonyms, but based on the context, they can replace each other. Thus, in the Lexical Relations and SEO Case Study, I have focused on these lexically important connections. A verb can be a noun, synonym, or adjective and adverb based on the word sequences.
And, not just the word, but word sense, and word role should be matched with the query patterns. The queries have to be clustered with two different phases to create a proper semantic content network. One is “verbs”, and the second is “nouns”. The first classification comes from the verbs, and it creates semantically connected behaviors. The second classification comes from the “nouns”, and it creates the connected outcomes. “Learning a skill”, and “improving a skill” are connected to each other. “Making money from that skill”, or “earning more by increasing the skill efficiency” are connected to previous verb and noun connections. Based on these connections, many granular contexts can be acquired and processed better than other websites do.
An Example Process for the Connections of Verbs for the Creation of Semantic Content Networks
For the project of IstanbulBogaziciEnstitu.com, the two different semantic content networks are created and connected to each other. Based on that, “entity-oriented search” has been used as stated and processed based on the BKMKitap.com SEO Case Study. The source context here is “course selling.” And, most of the time, the courses are bought by young people who want to process and further their careers and lives.
In this context, “learning an occupation”, and “earning money from it” are representative and canonical verbs and nouns. From here, “X Course”, and “Tips to Learn X”, “X Books, Podcasts”, and “X related occupations”, “X Occupation necessities”, “Universities for X Occupation”, “Salaries in X Occupation” are processed.
This type of source context requires becoming an authority for multiple different entities, but also search behaviors. For example, it is not good enough to be an authority only for “X”, but also for “courses”, “tips”, “learning”, “comparison of occupations”, and “career and salaries” contexts should be processed across the entire website. Thus, the entire website has to focus on two different things.
Concepts that encapsulate smaller members, such as occupations, or skills.
Verbs for these concepts encapsulate smaller verbs, such as “understanding”, or “grasping”.
This encapsulation is called “entailment” in lexical relations for the SEO case study.
In this context, two different semantic content networks have been created.
- Universities and Occupations
- Occupations and Salaries
The third semantic content network that hasn’t been published yet is to provide better contextuality, be way more relevant and drive more traffic for “courses”, and “definitional concepts”. For example, it is not good enough to process “programming occupations, universities, and salaries”, but also “programming languages”, or “programming glossary terms” should be done. When a source contains all this information, it becomes a topical authority on three main things.
- Education
- Career
- Education and Career-specific Concept Definitions
The third part is way more prominent for the course selling, and conversions. In terms of semantic distance, “X Course” and “X Definition” are closer to each other than the “X Occupation”. Semantic Distance and Similarity SEO Case Study explained the measurement of these concepts by explaining the importance of Definitions for SEO.
What is the prominence of Semantic Role Labels for Query Semantics?
Semantic Role Labels are the role specifications of words in a sentence based on their contribution to the meaning of the sentence. Search Engine Optimization with semantics and sentential NLP (NLP for sentences) are closely related to each other. A verb that is qualified and performed by an object in the sentence involves a predicate. Semantic Role Labeling works based on “predicates”. A predicate signals the prominence of an entity within a sentence and its effect on another thing with a role. Frames and FrameNet are relevant to the Semantic Role Labels in this context.
But, why is SRL important for Query Semantics? Without keeping it, it is too academic and diverse with other concepts such as frames, or FrameNet, let’s give a solid example, together. Entity-indexing, or re-indexing the existing documents based on the entities, involves the “SRL” and “predicates”. A semantic search engine organizes its index, and query processing methods based on the entity-attribute-value (EAV) database structure. To differentiate different contexts, and sub-topics from each other, the “EAV” requires “predicates”.
In other words, to be able to index the entities, and classify the different sub-contexts of these entities, a search engine needs to understand the prominence of “predicate” in the sentence, and these “triples” should be organized based on the “predicates”.
To classify the group of web documents for better index partitioning based on the entities, a search engine needs to classify the queries. If a search engine uses entity-attribute-value triples for index partitioning, it needs to understand the “verbs” in the “query semantics”.
Let’s dive into a couple of semantic role-labeling samples.
In the sentence “X runs the Y Company in Wall Street since 1971.”, the “X” is the subject, “Y” is the object”, and “run” is the predicate. From the SRL perspective, the “X” is the agent, “run” is the predicate and “Y” is the “theme”. If we go deeper, this sentence is represented below in the Semantic Networks.
The “attributes”, “date”, and “named entity Y” and its attribute-value pair, “type-company” pair are connected to the X, with the “run” predicate.
Imagine that the search engine has, 10000 queries from the query logs from different timelines that mention the “X”. And, 56% of these queries use a predicate such as “run”, or “function”, “govern”, “manage”, “directing” and so on. Search engine cluster all these queries based on the “X”, and “run(predicate)” relationship, and all the “subjects” can come based on the “theme” which is the company since the X’s main profile is a manager.
The Query Semantics section of this SEO Case Study has been written on 26 May 2022. The video below is from December 2022, it shows the continuation of the Holistic SEO Approach for Semantics, and Topical Authority methodologies.
w
An Example of Verb Connections in Neurons
Search engine imitates the human mind with their own perception, to guess our search behaviors. Thus, search engine algorithms and users are mind twins that feed each other. An algorithm might change how a user searches and an algorithm can be changed based on how the user searches for the same query.
Thus, let’s check how the search engine predicts the mid-string query, and end-string query refinements (auto-suggestions from the search bar), based on the predicates.
The predicate “increase”, directly brings “hormones”.
The predicate “decrease”, brings file types, and again the hormones, or anything in the blood (for the human body).
The predicate “melt” brings “foods”, and most of these foods are for “recipes with multiple steps”.
But, when it comes to the “freeze”, the “theme” is usually digital platforms or technologies.
Every predicate has a specific “theme” for its own possible context. And, there is a reason that after every predicate, search engines choose similar entities to each other such as hormones, programs, or file types. Every predicate includes one object and a subject with a theme.
Some Semantic Role Labels are below.
In reality, there are more than 32 different semantic role labels. A live example is below.
In this context, a search engine can create connections of entities based on the verbs, and verb effects. A loader and a loading process need to have specific types of causes, reasons, results, objects, and subjects around them. To understand how to choose the right verbs as canonical, and how to cluster another verb + noun phrase patterns around them, let’s check the “themes” of “words.
What are the themes of words?
Like verbs, every word, or word role has specific types of roles. When I say “ballerina”, and guess a century, the person might say “18th century”, or “21st century”, but the “image in the brain” changes based on the century. The theme of the word and other concepts around it changes based on our perception map. To summarize this topic, let’s use the “Vocabulary Word List by Theme from Word Bank”.
There are many themes for every word. And, let’s choose one of these things, such as “beach”. The words “barnacle”, “boardwalk”, “boat”, “crab”, “catamaran”, “clam”, “dock”, “dune”, “ocean”, “limpet”, “dive”, “swim”, “hat” and other types of words are from the same theme. But, if we add another word here, such as “Wall Street”, the theme has to change suddenly. And, “Understanding the verbs of life” is important to understand how to consolidate these context terms and vectors. Because all these thematic words come strongly from the “verb” connections. Nouns have to be affected by the verbs, based on a purpose, and cause-result relationships. And, these help in creating Semantic Search Patterns for possible search activities.
Understanding the FrameNet for Semantic Search
As in Semantic Role Labels or the Themes of Words, FrameNet is a meaning organization based on patterns from human life. FrameNet focuses on “verbs” to understand the semantically dependent results, or the tools and other parts of the situation. Professor Charles Filmore has started the FrameNet Project to explain the conceptual connections between the patterned situations.
For example, let’s choose three different verbs.
- “Cook”
- “Run”
- “Move”
For all three of these verbs, the “agent” needs specific types of agents and connections.
- For the “cook”, a “heat source”, “heating tool”, “food recipe”, and “calorie and nutrient” connection is necessary.
- For the “run”, it requires more context, but for all these sub-contexts, “a company”, or “a destination”, a methodology or a destination is required.
- For the “move”, the “moved object”, “moving tool”, and “moving destination”, is necessary.
All these connections come from the verbs. And, a search engine seeks these connections and framed patterns on the web documents to understand their classified categories and possible sub-contexts.
- If a topic is about “losing weight”, using the word “dietitian” might be better than “doctor”.
- If a word has 5 different possible themes, using a most specified and narrowed term first would be better to specify the context.
- If a verb contains a specified framed pattern, giving the steps or stages, parts and types of these patterns from the first sentence would be better.
- If a document is not organized based on the semantic search patterns, the document, and document network should be optimized and re-organized based on the semantic search principles.
FrameNet involves different types of syntactic roles for every sentence, or sentence part, on the open web. The FrameNet involves the “subcategorization frames”, and “diathesis alternations”. Diathesis alternations involve the change of the verb as a usage or phrase. As I mentioned before, the same verb can mean two different things, or the same verb can be used in two or more different ways for meaning the same thing. These alternations help a search engine to compare, or match the competitor, and alternate web pages for specific queries. The subcategorization frames involve the co-occurrences of words in different proximity for changing the context of the frame. Lexical Units are necessary to understand the word-sense disambiguation and to choose the right subcategorization frames.
FrameNet usage sample from NLTK is below.
The example above is from NLTK Official Documentation. NLTK is heavily used by Google Engineers since it is a basic and fundamental Python Library for NLP. The example above shows the usage of the different frames. An example has been chosen for “criminal”, and the chosen sentence explains “criminal investigation”.
Lexical Units from the chosen frame show the words and components like “offense”, “time”, “suspect”, “source_of_legal_authority” and more things. It is “arrest”, and a “child”, or “subframe categorization” of the “Criminal Process”. Thus, all the verbs and themes of words are pre-determined. In this context, semantic search behavior can have certain types of aspects. In my Semantic SEO for News Sources article and tutorial, I explained how specific situations and patterns can be used to create semantically connected and relevant news article patterns.
Similarly, the “verbs” and their connections, or any possible “noun” that can come after the specific verb, and their connections are helpful in the creation of different types of Semantic Content Networks. Semantic Content Networks focus on semantic search behaviors to complete all possible semantically connected concept query sessions.
Differentiation of the Lexical Units for Better Verb and Noun Themes
Verb and Noun themes involve certain types of semantic behaviors. Usually, in knowledge representation, these are called “frames”, frames and FramNet shouldn’t be confused with each other. Lexical Units are the sub-parts of FrameNet. FrameNet Lexical Unites can be hyphenated words, idiomatic phrases, or single-term words. But, these words might have different “verb” versions.
For example, the verbs “bake”, “bakes”, “baked”, and “baking” lemmas might mean applying heat, cooking, or absorbing the heat. Different nouns can use the same verb and its same version, as below.
- The potatoes have to be bake for more than X minutes.
- The aubergine has to be baked for more than Y minutes.
- The courgette has to be baked for more than Z minutes.
The X, Y, Z are connected to a specific entity attribute of the potato, eggplant, and courgette. They are all entities of the same type. And, “baked” is used for “absorbing the heat” for a longer time. A semantic network and semantic content network can be created based on these connections. And, if we use the “bake” verb for the meaning of “applying heat”, the sentence structure will change, and it will need to catch the query perspective.
- The chef needs to bake potatoes for more than X minutes.
- The chef needs to bake the courgette for more than Y minutes.
- The chef needs to bake the eggplant for more than Z minutes.
The Semantic Role Labels changed. If the queries contain “natural language” by saying “How do I bake potatoes….”, then, this type of lexical unit and SRL are better for matching the query terms, and their possible answer format.
SEO Case Study Examples for Semantic Search and Web
TheCoolist SEO Case Study from Use of Predicates Point of View.
The expanding topical map SEO Case Study explains the topical map expansion logic and reasoning of TheCoolist.com, but it doesn’t explain the predicates’ contribution to topical map creation and expansion. TheCoolist focuses on any kind of listing or set of entities. Any kind of listicle for a specific type of plural entity type is used to be compared to each other and also suggested to the users. It means that the source is a “broad topical source” without a particular consolidation, but it is an authority for certain types of query templates, such as “X Amount of Ys with a superlative word”. Adjectives like “best, highest, smallest, top”, and nouns like “dogs, cars, questions, myths, or formulas” for certain types of contexts such as “lifestyle, well-being, decoration, or beauty” are connected to each other. Thus, topical authority is not just about topics, but it is about patterns of queries, and documents that match them. The predicates for every listicle have the contexts of “compare, learn, remember, review, examine, think about, inspect visually, textually, compare in tables, or infographics”. Along with these, there are uncommon predicates for certain types of listicles such as “improve a skill” for the listicles like “10 ways of learning X”, or “20 methods to do X”, and “socialize better” for the listicles like “20 questions to ask to Y”, or “40 ways of telling P to C”, there are other listicles for a career such as “10 tips for X occupation”, and “30 ways of asking for a salary”, etc. If you think carefully about predicates, all these “ways to do X”, or “tips for P” involve certain types of aspects that can be connected to specific types of contexts. These contexts can be used for “query-focused vocabulary configuration” and “grouper semantic content networks” by closing the topical gaps that are explained in the Entity-oriented search SEO Case Study. Thus, the topical map focused on “personality types”, and “personality types” go for celebrities. And, you can solve the next puzzle piece from here. Semantics do not have a proper SOP, they have a thinking game that takes its rules from the human brain, and searches engine engineer conditions.
The results are above and below.
The Snuffstore.de SEO Case Study from Use of Predicates Point of View.
Snuffstore.de is explained in the Expansion of Topical Maps SEO Case Study, and Lexical Relations, but we didn’t focus on predicates for creating the semantic content networks. The semantic content networks help connect predicates and nouns with certain attributes to signal the context. The topical map of the project is explained for expansion logic in the respective SEO Case Study. Here, we explain how predicates help with it. The Snuffstore focuses on e-cigarette products, but it doesn’t use the predicates enough that can come with this specific entity such as “smoking”, “consuming”, “using”, “draw on”, “vapor”, “smog”, “fume”, “light up”, “smolder”, “reek” and others. “smoking” is connected to the “harming health”, or “relaxing mood”, and “producing”, “manufacturing”, “comparing”, “buying”, “selling”, “changing” are other related context terms. The other predicates such as “steam”, “brume”, “murk”, “fog”, “mist”, “miasma”, “pip”, “cloud”, “soup” should be used for other relative nouns beside the e-cigarette with specific adverbs, and sub-types of these set of entities to cover everything. The definitive and strongly connected components come with the predicates that are used for the same attribute-context pairs with the nouns which help for the expansion of the topical map. The “theme of words” is another topic that will be explained in the future, further.
27 Articles Website SEO Case Study from Use of Predicates Point of View.
Out of respect for the client, I still didn’t explain the name of this specific website, but using 27 articles to increase the traffic over 10x, and take organic queries, and rankings from global authorities such as WebMD, Healthline, and Medicalnewstoday is possible without backlinks, as is proven. I have videos that record my edits on these articles, I can share the name and further details in the future, but for now, representatively I will explain, in general, how the predicates helped us to understand and leverage semantic search for semantic SEO.
The specific project, in my opinion, will lose traffic in the future, as I warned other projects in the beginning, pacifism is another principle that is broken for Topical Authority methodology, in other words, momentum is lost.
The predicates helped this specific website to create and expand the topical map based on the Source Context. The Source Context should be read from the Expansion of Topical Map SEO Case Study. The Source Context focuses on a certain type of health-related and luxury product at the same time. Thus, “consuming X”, and “using X” are connected with different layers of the same contextual domains, such as “consuming X in the morning,” “consuming hot X, “Consuming hot X”, or “Consuming X before Y”, and all these context qualifiers are connected to a certain query template. The verb “consume” is connected to the other entailing predicates such as “use”, “prepare”, and “understand.” The entailed predicates are way more specific, thus, giving them would expose the project’s industry. For now, this section stops here. And, this is a good example of how the predicates give context and topical maps. With that said, entailing verbs are verbs that entail multiple meanings, processes, or repetitive and one-time actions, while entailed verbs are more specific and contextually explicit.
Sunnyvalley.io SEO Case Study from Use of Predicates Point of View.
Sunnyvalley.io is an SEO project that leverages AI content writer assistants for increasing brand awareness, content production speed, and brand building. Semantics and Topical Authority help build brand authority and identity. Sunnyvalley.io Topical Map and Semantic SEO practices for brand building and organic search performance improvement are explained in terms of the SaaS SEO Guide on the Koray Tugberk GUBUR YouTube Channel, along with the JookSMS SaaS SEO example.
The predicates in the topical map of Sunnyvalley.io are not explained before, thus, this section doesn’t focus on the lexical relations or expansion of the topical map, it focuses on the predicates’ role in it.
The Sunnyvalley.io topical map and semantic content network work with knowledge base creation, and the knowledge base creation is about using the “related” search operator to have the link graph, and Google’s classification algorithms as a partial output. In this context, the most important predicate and noun pairs are “provide network security”, “learn network security”, “compare network security technologies”, “buy network security service”, “use network security program”, “have network privacy”, “prevent network hack”, “understand network security threats”, “strengthen the company network security”, “use network security principles”, “leverage network security”, “grasp network security types”, “secure the network”, “breaching data center”, and more. All these predicates are united with different phrase taxonomies such as “network security program|technology|hack|threats|types|service”. All the phrase taxonomies make the query networks and conditions more explicit and narrowed with certain context vectors. Phrase taxonomies are processed in the Semantic SEO Course further.
Link
5. NASDAQ SEO Case Study
NASDAQ SEO Case Study is processed in Lexical Relations and the Expanding Topical Map SEO Case Studies by providing the NDA that discourages me from sharing the web source name, and the video tutorial for NASDAQ SEO has been processed as below.
The specific section focuses only on the predicates and their connections. The predicates that form the specific semantic content network with some phrase taxonomies are “print 3D X”, “Print Prototype C”, “Mold P”, “Cutting V”, “Lasering W Material with P Machine”, “Using R Technology for W Type of 3D Printing”, “Providing 3D Printing Service”, “Understanding Heavy Metallurgy”, “Comparing X material to Y Material”, “Know C material”, “Know P Inventor”, “Compare C Machine to P Machine”, “Configure X Machine for P Cutting”, “Learn T Cutting for P Material with Z Machine”. The predicates here provide “context signifiers” such as “for”, “with”, and “via” together with the phrase taxonomies. The “context signifiers” are processed further in the Information Responsiveness Book and SEO Case Study in 2023.
6. EB5Brics.com SEO Case Study from Use of Predicates Point of View.
B5Brics.com is a project that I managed with the help of certain types of search contexts’ coverages because EB5brics focuses on a certain type of Visa, and visa application owners such as investors. Thus, it is relevant to the two pilot projects that I managed before, Istanbulbogazicienstitu and Vizem.net. Because, both of these pilot projects were able to acquire their growth thanks to the structured and disciplined mindset that focuses on semantic and contextual coverage with information richness and quality, until they decrease the quality with topical map distortion for small queries, and leave the semantic content networks uncompleted. Thus, the EB5Brics is a good Visa website example as a criticism for Vizem.net. Because, Vizem.net distorted the topical map by opening web pages for micro-queries by diluting the Ranking Signals, and dividing PageRank, along with the relevance and new articles didn’t protect the quality of the previously published articles. Thus, the EB5brics provides a better consolidation, but it focuses on “Investment”, “Citizenship”, and “Visa” connection at the same time along with the “Immigration”, and predicates come from these intersections. These predicates are “go”, “travel”, “settle down”, “invest in”, “immigrate”, “apply”, “collect”, “provide”, “move in”, “flying”, “transit”, “tour”, “trip”, “voyaging”, “touring”, “running (business)”, “founding (company)”, “registering (brand)”, “approving”, “learning (culture|laws|food)”, “managing (business, family)”, “parenting (in a different…)”. All these predicates are connected to each other with certain combinations, and query semantics, along with contextual search understanding help Semantic SEOs to connect entities and attributes to each other properly.
7. From 0 to 1,200,000 Organic Clicks SEO Case Study from Use of Predicates Point of View.
The specific project is protected due to semantic relevance and responsiveness thresholds, and the client’s simplistic approach, which allows possible competitors to create new cornerstone sources easily in comparative ranking logic. Thus, the information that is given here in terms of predicates, and their purposes and semantic search connection is reflected to explain the perspective while protecting the project. The main contextual and entailing predicates that are used “know X”, “learn C”, “calculate P”, “use V”, “Learn meaning of X on C”, “Understand nature of P on Z”, “Indicating X”, “Representing X”, “Feeling X”, “Connecting representation of X to feeling of X”, “Involving P and C based on E condition”.
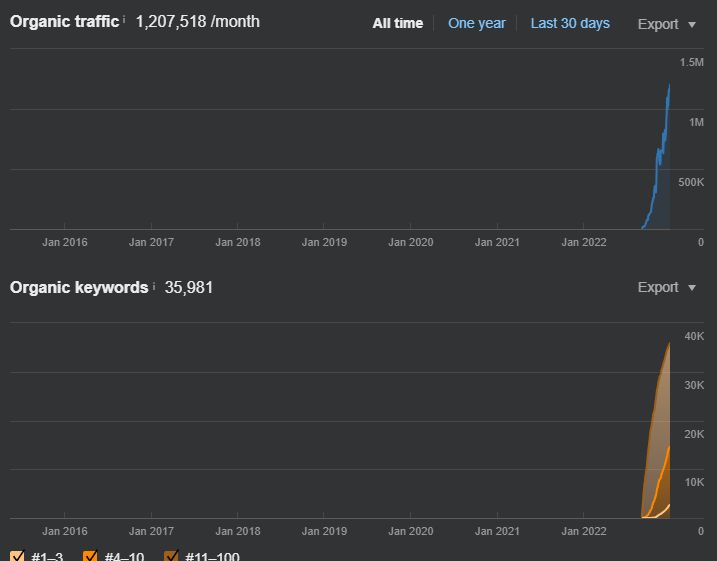
What is Frame Semantics?
Frame semantics is an influential theory of meaning that has been gaining traction in the field of linguistics and natural language processing. It proposes that words are understood not only by their dictionary definitions, but also in terms of their relationships to other words within a frame. A frame consists of structured networks or concepts and relationships which provide context for understanding the meaning behind any given word or phrase. For example, when considering the word “eat” one might consider related concepts such as food, hunger, restaurant, and cutlery; all these elements form part of its overall semantic meaning within this particular frame.
The use of Frame Semantics can be incredibly helpful when attempting to understand more complex sentences, where multiple frames may interact with each other to create an even richer understanding than just looking at individual components alone could provide us with. Additionally, it can help computers better comprehend human language by providing them with deeper insight into how people communicate naturally using contextual clues provided through frames rather than simply relying on literal translations from dictionaries alone. Furthermore, it helps us recognize patterns in our speech, so we have a greater ability to identify potential miscommunications before they occur, leading to improved communication between humans (and machines).
In conclusion, Frame Semantics is an invaluable tool for helping both humans and machines better understand spoken language due its ability to capture contextual information from multiple sources simultaneously, allowing for increased accuracy when interpreting meanings associated with certain words/phrases over traditional methods like dictionary definitions alone would allow us to achieve otherwise.
Concept | Definition | Historical Origin |
Frame semantics | A theory of meaning that explains how language is used to convey concepts and ideas by evoking certain frames or mental structures in the listener or reader. | Developed by linguist Charles J. Fillmore in the 1970s. |
Natural language processing | A field of computer science and artificial intelligence that deals with how computers can understand, interpret, and generate human language. | Developed in the 1950s as a subfield of linguistics and computer science. |
Semantic search | A type of search engine technology that uses meaning and context to understand the intent behind a search query, and to provide more relevant results. | Semantic search technology has been developed since the late 1990s but has become more widely used in the 2010s with the advent of machine learning and natural language processing techniques. |
Semantic web | A vision for the future of the World Wide Web, in which information is more easily understood and shared by computers, and can be linked and combined in new ways. | Proposed by Tim Berners-Lee in the late 1990s. |
Semantic SEO | The practice of optimizing a website or web page for semantic search engines, in order to improve its ranking and visibility. | Developed in the 2010s as a response to the increasing use of semantic search technology. |
Formal semantics | A branch of linguistics that deals with the formal analysis and representation of the meaning of words and sentences in a language. | Developed in the 1950s as a way to formalize and analyze the meaning of natural language expressions. |
Topical authority | A measure of the expertise or credibility of a website or web page on a particular topic or subject. | Developed as a way to evaluate the quality and reliability of information on the web. |
Discourse integration | The way in which a particular word or phrase is used within a larger discourse or conversation, and how it relates to the context and overall meaning of the discourse. | Developed in the field of frame semantics in the 1970s. |
FrameNet | A database of frame semantics that aims to provide a comprehensive and systematic description of the meanings of words and phrases in a language. | Developed by linguist Charles J. Fillmore and his colleagues at the University of California, Berkeley in the 1990s. |
Context term | A word or phrase in a search query that provides context or background information about the topic being searched. | Developed as a way to understand the intentions and needs of users in search queries. |
Knowledge domain term | A word or phrase in a search query that is specific to a particular field or area of knowledge. | Developed as a way to understand the expertise and interests of users in search queries. |
Answer term | A word or phrase in a search query that indicates the type of information or result that the user is seeking. | Developed as a way to understand the intentions and needs of users in search queries. |
Information retrieval | The process of finding and retrieving information from a collection of documents or data. | Developed in the 1950s as a way to manage and access large collections of documents and data. |
Information extraction | The process of automatically extracting structured information from unstructured data sources, such as text documents or web pages. | Developed in the 1980s as a way to automatically extract and organize data from large collections of documents and data. |
Why is Frame Semantics relevant to Semantic Web?
Frame semantics can be used to create these ontologies in a way that is more intuitive and easier for computers to understand. For example, if you wanted to develop an ontology of different types of food, you could use frame semantics to represent the relationships between different categories such as “fruits,” “vegetables,” and “meats.” This would allow a computer system to better comprehend the meaning of the data within each category.
Finally, frame semantics can help with natural language processing on the Semantic Web by providing context for understanding words or phrases in their proper context. By using frames that include concepts related to a particular word or phrase, it becomes easier for computer systems to recognize when certain words are being used correctly or incorrectly. For instance, if someone entered “apple” into the search engine on a website about food items, then frame semantic technology might be able to determine whether they were looking for information about apples (the fruit) or Apple Incorporated (the company).
Frame Semantics provides powerful tools which enable machines to interpret data from web resources accurately and efficiently, making it a relevant tool in the development of The Semantic Web applications
What is Semantic Web?
The Semantic Web is a revolutionary vision for the future of the World Wide Web. It involves annotating data with machine-readable metadata so that computers can process and understand its meaning. This will enable them to better support human activities such as search, data integration, and knowledge management. In order to achieve this goal, several technologies and standards are employed, including Resource Description Framework (RDF) and Web Ontology Language (OWL). These allow different sources of data to be connected more easily by describing them using common concepts or relationships; thus creating an interconnected view of the world which is more comprehensive in nature.
Philosophers have long argued that understanding our environment requires us to interpret what we observe through language – something only humans can do effectively at present time, but could potentially be achieved by machines in the near future if they are able to comprehend semantic information from web documents accurately enough. The Semantic Web provides a framework for achieving this goal as it allows computers not only to access large amounts of well-organized structured information but also gain insights from unstructured content such as natural language text on websites or social media platforms where most people get their news.
What is Semantic Search?
Semantic search is a contextual information-seeking methodology on web search engines that provide meaningful associations with human-language processing and understanding capacity by improving the accuracy and relevance of search results. By understanding the meaning, need, related and possible search activities behind a web search query of an intelligent searcher, semantic search engines are able to provide more targeted and accurate results that involve frames that are shaped around the semantics with predicates, and nouns, than traditional keyword-based searches. Semantic Search helps search engines to understand, cluster, and match the users with better topically authoritative sources with local, and contextual relevance. The semantic searcher requires authoritative and accurate information sources with proper service-providing platforms for their search needs, thus search engines need semantic search capacity for understanding the purpose, layout, components, ontology, taxonomy, entities, attributes, values, predicates, frames, contextual coverage and richness of the documents, and sources’ overall expertise signals that own the documents. Semantic search can’t be seen only search with meaning, it is a search with context for expert sources of these contexts that have accurate, quality, and unique information for the patterns, and frames for the specific contextual domains.
The semantic search involves finding patterned behaviors of the companies, and users, for certain contexts. Thus, web search engines started to construct their indexes for query templates, document templates, and search behavior patterns. FrameNet, predicates, and triples in the form of subject-predicate-object are relevant to the semantic search. Semantic Web is the World Wide Web that is shaped according to semantic search behaviors and principles. The semantic Web involves semantically coded websites in terms of design, layout, content, and user needs. A semantically optimized website has consistent, logically constructed, strongly connected associations between topical terms and patterns in the form of an information graph. The information graph of a source becomes an authoritative source effect for the web search engine’s corroboration of web answers’ algorithms for finding the truth, factoids, and question-answer repositories for different knowledge domains.
What is FrameNet?
FrameNet is a powerful way for linguists and natural language processing researchers by providing an organized structure of concepts and relationships FrameNet makes it easier to identify the meaning of words in the context which allows for more accurate machine translation text analysis dialogue systems information extraction from text and other applications that require understanding language at a deeper level than just word-by-word translations the FrameNet database is constantly being updated with new frames as well as annotations to existing frames researchers can use the data provided by FrameNet to develop better models for natural language processing tasks such as sentiment analysis or question answering systems. The research community has also created tools that allow users to quickly and easily search through the database in order to find relevant examples or explore related frames without having to manually comb through all, 13000 entries in the database. FrameNet provides an invaluable resource for those researching or developing applications related to natural language processing by giving them access to structured networks of concepts that provide insight into how words are used in different contexts within sentences it is constantly updated with new content so researchers have access up-to-date information about frame elements and their relationships when working on their projects.
How does Frame Semantics help for Semantic SEO?
Frame Semantics involves FrameNet database, and beyond with the conceptual patterns that are shaped around the predicates, and the verbs. Semantic SEO with Frame Semantics put the predicates (verbs inside sentences) at the center of the meaning to shape the contextual flow of the document. Frames with semantics involve triples with predicates, objects, and subjects. With the correct and contextual predicate, the semantics relies on finding the correct object and subject, which is followed by another connected frame. The connections of the frames help for creating semantic networks, which is a methodology of knowledge representation. Frames and Semantic Networks are two different contexts and meaning representation that encapsulates certain information. Frames have rows and columns that are connected to each other. A frame is connected to another frame with certain objects, subjects, and predicates. Following the objects with attributes and values help in using the Entity-Attribute-Value data structure, and using the predicates helps in using the Subject-Predicate-Object data structure. For both of the data structures, using the Frame Semantics, and context terms that encapsulate answers for certain knowledge and context domains is needed.
Why are Semantic Content Networks relevant to Frame Semantics?
Semantic Content Networks are found by Koray Tugberk GUBUR for World-Wide-Web improvement in terms of semantic search, and semantic search engine optimization. Semantic Content Networks are semantic networks in the form of textual and visual content. The content network that is constructed with semantic connections is transferred easily into the data in the form of semantic networks, frames, and information graphs. Thus, it is easier for search engines to process, evaluate, and associate with constructed indexes to satisfy web search engine users. The semantic content networks are connected to Frame Semantics because semantic content networks leverage predicates to understand contextual borders and nouns for understanding topical borders. The unification of entities with predicates creates topical maps for topical authority improvement with the help of search engine trust and historical data.
What is a Context Term in NLP for Frame Semantics?
In natural language processing (NLP), context terms are words or phrases that provide additional information or context about the meaning of another word or phrase. Context terms can be used to disambiguate the meanings of words and phrases, helping NLP systems understand them more accurately in tasks such as text classification, information extraction, and machine translation. For example, consider a sentence like “I caught a large bass while fishing yesterday”; without any context term, it would be difficult to determine whether “bass” is referring to a type of fish or something else entirely. The contextual term “while fishing yesterday,” becomes clear that this particular instance refers specifically to an aquatic creature rather than other possible interpretations.
Contextual terms can also help machines better interpret figurative languages such as metaphors and idioms by providing additional hints about their intended meaning from within the same sentence structure they appear in. By taking into account both literal meanings derived from individual words and contextual clues provided by surrounding text elements such as adverbs and adjectives, NLP systems can make more informed decisions when attempting tasks related to understanding natural languages written by humans. Using multiple types of contexts – including semantic contexts, which take into consideration how different parts interact with each other – further enhances accuracy for many NLP operations.
What is Word Sense Disambiguation for Frame Semantics?
Word sense disambiguation (WSD) is an important process in natural language processing and computational linguistics. It involves identifying the correct meaning or sense of a word when used in a particular context. This process allows computers to better understand and interpret human language, which can be very useful for tasks such as automatic translation, question-answering systems, text summarization, and more.
In frame semantics – an approach to linguistic analysis that focuses on how words are used within different contexts – WSD can be used to determine which frame is most relevant for a given word or phrase based on its usage in the sentence it appears in. For example, consider the word “bat.” Without any context clues it could refer to many things: flying mammal; wooden club; electronic device etc., but if we use this same term within the context of “The baseball player swung his bat at pitch,” then we know that our target concept must relate back to hitting balls with clubs rather than anything else mentioned above.
What is an Answer Term in NLP?
Answer terms are a valuable tool in natural language processing (NLP) applications, allowing systems to identify and extract relevant information from text or generate appropriate responses to user queries. In NLP tasks such as information extraction, question answering, and text summarization, answer terms can provide the system with the necessary data to accurately respond to user inquiries.
For example, consider the sentence “The capital of France is Paris” – here “Paris” would be identified as an answer term that provides an accurate response to a query asking “what is the capital of France”. Answer terms allow NLP systems to process this kind of query quickly and efficiently by extracting specific words or phrases from sentences in order to determine which one contains the correct answer.
In summary, understanding how answer terms work within natural language processing applications can help ensure that your system responds accurately when faced with questions related specifically answered by certain words or phrases found within texts. By recognizing these answers early on, you can further enhance your application’s ability to make sense of large amounts of data quickly and effectively, while also providing users with the reliable results they need.
What is an Answer Term in Frame Semantics?
Frame Semantics involve answer terms to determine which frame and frame elements involve the specific answer term for which context with what works, and disambiguated senses. It involves the use of frames, which are collections of related concepts and associated elements that help define how a particular concept is used in natural language. Each frame includes example sentences, annotated text, and answer terms – words or phrases that provide the answer to a specific question or query associated with the frame.
For example, consider a frame for “animals” – it might include elements such as species, habitat, and diet; along with sample sentences showing how these terms are used in context. In this case, an answer term could be “carnivorous” when asked about the diet of lions; providing an accurate response to user queries on animal diets without requiring manual input from humans each time.
Answer terms can also be useful in tasks such as information extraction by allowing systems to more accurately identify relevant data within large amounts of unstructured text (such as news articles). By understanding what questions users may ask based on their knowledge base (the set of frames available), they can quickly extract answers even if they don’t appear explicitly stated within documents.
What is a knowledge domain term in NLP?
Natural language processing (NLP) is a rapidly growing field, and knowledge domain terms are an important component of many NLP tasks. Knowledge domain terms are words or phrases that belong to specific areas of expertise or knowledge, such as physics, biology, engineering, etc. These terms can be used in text classification systems to identify the relevant subject of a given piece of text or query. They can also help information extraction systems better understand the context and content within documents, so they can extract more accurate data from them.
In addition to these applications for NLP tasks like text classification and information extraction, knowledge domain terms have been found to improve machine translation accuracy as well. By providing additional contextual clues about the source material being translated into another language—such as identifying certain scientific concepts—machine translation models become much better at accurately translating complex texts between languages while preserving their original meaning. For example, if a web search engine user asks a question about underwater plants, and ecosystems, the terms that do not appear in other knowledge domain terms, predicates, frames, and associations have to appear in the quality answers. Thus, if an answer term appears in a certain knowledge domain, it is a knowledge domain term for natural language processing and semantics.
What is a knowledge domain term in Frame Semantics?
Frame semantics involves assigning meaning to words or phrases in order to provide additional context and understanding within a particular knowledge domain.
A key component of frame semantics is the concept of “knowledge domain terms” – words or phrases that belong to a specific area of expertise, such as particle physics. These terms are associated with frames or collections of semantically relevant linguistic elements such as subatomic particles, experiments, and theories which give them additional meaning within their respective domains. For example, in particle physics, we might use “Higgs boson” or “Standard Model” as knowledge domain terms that help us understand this subject better by providing more context around it than would be available without these important concepts being identified explicitly.
Knowledge domain terms can be used for various tasks related to natural language processing (NLP), including identifying relevant information from text sources and generating appropriate responses based on user queries. Knowledge domain terms and Frame Semantics help web search engines to cluster documents and match them with query clusters with certain contextual layers. Contextual layers are the hierarchy of contextual domains according to the dominant context, paths of thinking patterns in the contextual domain that appear in the search queries, and document statistics with linguistic components. For example, a document that involves the cause-effect relation for two different associated entities with certain attributes is classified and labeled differently than a document that involves the shipment of X and Y together with use purposes. Thus, the knowledge domain terms will slightly change in a way that is reflected in the document statistics with linguistic signatures. The context qualifiers, and signifiers such as “shipment condition of X” might be caused by the “heat of Y”. If Y is a source of heat for X, it might affect many other attributes and their values for X in different types of frames.
Why is frame semantics relevant to semantic role labels?
Frame semantics is relevant to semantic role labels because they provide insight into how people use language to convey meaning beyond what can be expressed through individual word meanings alone. Semantic role labels help identify roles or functions of words and phrases within a particular frame by describing their relationships with verbs or other parts of speech in sentences such as subject, object, location, etc. This helps us better understand why certain combinations of words have specific connotations; for instance, “The cat chased the mouse” has an entirely different connotation than “The mouse chased the cat” even though both sentences have similar grammatical structures but differ only slightly in terms of semantic roles assigned to each term (cat being agent while mouse being patient). By analyzing these types of relationships using frame semantics principles, linguists gain valuable insights into nuances associated with particular lexical items which would otherwise remain hidden from view without this type of analysis approach.
In conclusion, frame semantics provides a useful tool for identifying meaningful patterns among various syntactic constructions, allowing us to uncover subtle distinctions between seemingly identical expressions. Additionally, the frame semantic approach allows us to gain deeper insights into underlying mechanisms behind the construction of complex sentence structures, thus providing invaluable information about the way humans communicate effectively using natural languages
What is Discourse Integration for Frame Semantics?
In frame semantics, discourse integration refers to the way in which a particular word or phrase is used within a larger discourse or conversation. It involves examining how the word or phrase relates to the context in which it is used, as well as how it contributes to the overall meaning and structure of the discourse.
For example, consider the word “dog.” In a conversation about a pet store, the word “dog” might be used in a way that is closely tied to the frame of PETS, evoking ideas about ownership, caretaking, and companionship. In a different conversation, such as one about a guard dog at a government facility, the word “dog” might be used in a way that is closely tied to the frame of SECURITY, evoking ideas about protection, vigilance, and alertness.
Last Thoughts on Semantic Search for Semantic SEO
The Understanding the Verbs of Life article focuses on how an SEO improves his/her own talents to understand semantic content networks, and linguistic structures. The second website sample of the Semantic Content Networks SEO Case Study will be updated after publishing the third semantic content network. Based on the new results, the teachings from this SEO Case Study Update will be taught further.
Verbs, nouns, and phrase patterns are used to structure the entity-oriented search engine indices. A search engine can structure its own understanding of queries, based on phrase patterns, query patterns, predicates, and triples. A triple with semantic role labeling can help a search engine understand the lexical units, and themes in the content further for authenticity, accuracy, and comprehensiveness. Semantic Search Behaviors and Patterns require understanding the linguistic semantics and query semantics further. By focusing on only the verbs, and their nature, we can create more useful, and helpful context terms, vectors, and context consolidation for the search engines.
See you on the new SEO Case Studies.
- Sliding Window - August 12, 2024
- B2P Marketing: How it Works, Benefits, and Strategies - April 26, 2024
- SEO for Casino Websites: A SEO Case Study for the Bet and Gamble Industry - February 5, 2024
I believe I will rank really higher with this
Fantastic! Every time I read it, I understand it more 🙂 L
Thank you Leanne.
Hello Koray,
This article opened my eyes on many things. Thank you.
Are these concepts applicable for other Search Engines (yandex.com is the one I’m curious about the most).
Thank you
Hello Dmitry,
Thank you for your kind words. Yes, all these concepts are applicaple for other search engines including Yandex, or Microsoft Bing, because most of the search engines started to be more and more semantic for over a decade, thus semantic SEO is universal for search engines.
There was no way I could afford your course. So, I’ve started reading your content, finally, and I think your words are very much understandable from where I am standing. The reading will be continued. Every day is a new day, a new content and some new concepts. Good Luck to me.
And Koray, thank you for the long, painful, eye-stressing, no time for pissing 10k+ word journey. It is very insightful. I obviously took notes, but I had to re-read some passages for how long or how many times I can’t remember. Still, the piece is worth the time. Thank You. I hope the World acknowledges your work very shortly.
Hey Muhymenul,
Thank you for your kind words, and comment. How about we gift the course to you? We are always open to friendly, and patient learners to support our abundance mindset.
Yetersiz ingilizcemden dolayı çevirerek okudum, tekrar tekrar okuyacağım . Bill Slawski’ nin blogundan bir şekilde senin çalışmalarına ulaştım. Umarım bu çalışmalarını Türkçe olarak da yayınlarsın , çeviri ile ilerlemek gerçekten zor.
May the semantic journey begin.
Welcome!
I have been doing SEO since 2016, applied many tactics, and failed many times. Your concepts and research are amazing. I learn from your free stuff and it’s very helpful to apply your concepts on my websites. You are providing this level of knowledge freely, literally amazing. It takes time to absorb this type of knowledge but I am still learning your concepts, I can’t afford your course but your free stuff is also worthwhile. keep sharing.
Thank you, Sibtain!